Why Generative AI is the Worst Form of Shadow IT
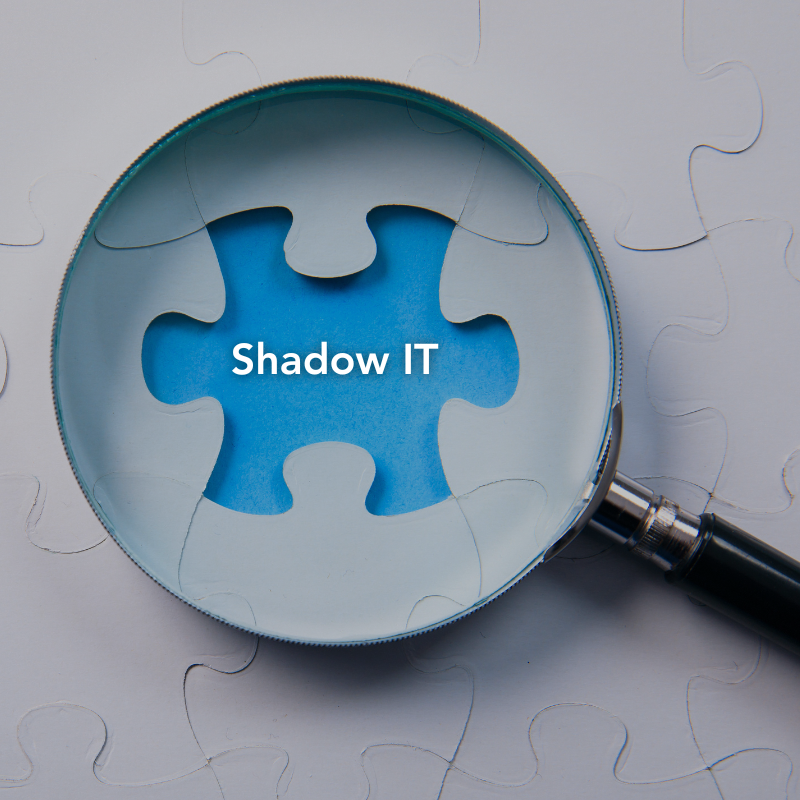
IT is the nexus for most organizations when it comes to all technology-related requests and implementations. Yet, as organizations grow, IT gets bogged down in these requests, which take longer to fulfill properly because they require managing security, privacy, and overall architectural concerns. Rather than waiting, some teams take it upon themselves to implement solutions independently, sometimes for temporary purposes and others as long-term solutions. This practice, otherwise known as Shadow IT, is so common that 42% of an average company’s IT applications comprise these uncontrolled and unmanaged solutions.
By existing outside of standard procurement and management channels, these shadow systems may be improperly patched or lack necessary security controls, making them easier targets for attackers. However, active attackers are not the only risk, as misconfigurations may simply expose sensitive data or open easy pathways, allowing even casual or internal threat access.
The Rise of Shadow AI
Shadow IT doesn’t just exist in traditional technology. It extends out into newer technologies such as Generative AI. Unsanctioned AI deployments within organizations, or Shadow AI, can be just as dangerous as any other IT. These systems often require vast amounts of data, including sensitive company information, which heightens the risk of security breaches if not properly managed. Detecting and monitoring these AI systems becomes a formidable task as they may exist as cloud applications, hosted 3rd party tools, or run locally by a team, expanding the possible search space.
Unauthorized AI tools can overburden IT infrastructure, leading to inefficient resource utilization and potential system disruptions. These tools often require substantial computational resources to process data, leading to competition with authorized applications and services.
An increased demand in AI can lead to:
- Network congestion
- Reduced availability of critical resources
- Potential system disruptions
Over time, the cumulative impact of unauthorized activities can result in:
- Degraded system performance
- Increased maintenance costs
- Potentially prolonged downtimes
- Severely affecting overall operational efficiency and service delivery
By operating outside official channels, Shadow AI poses substantial compliance risks. These systems bypass the stringent compliance standards required in regulated industries, leading to potential legal and financial repercussions. Without governance and comprehensive monitoring tools to manage these hidden AI operations, aligning them with internal policies and external regulatory demands is impossible.
Data Privacy and Security Risks
Regardless of its benefits, Shadow AI brings significant data privacy and security risks to address. At the very least, it creates an unidentified and unmanaged attack surface. This is a considerable issue considering the variety of sensitive data—financial, personal, and proprietary—commonly used in AI training, which, if compromised, can lead to severe repercussions.
The long-term storage of data presents additional challenges, as it must be protected against evolving security threats over time. Utilizing third-party data introduces further vulnerabilities and compliance issues, emphasizing the need for stringent data management practices.
The opaque nature of AI algorithms complicates incident management, making it difficult to respond effectively to security incidents. Because Shadow AI is unmanaged, proper incident response controls are impossible to develop. This amplifies the risk that an incident or breach will go unnoticed until it is too late or has a greater impact.
Understanding Governance & Compliance Challenges of Shadow AI
Deploying generative AI within enterprises presents significant governance and compliance challenges that underscore the need for rigorous oversight mechanisms. Core to these challenges is the establishment of TRISM principles—Trust, Risk, and Security Management—which are critical for ensuring trustworthiness, fairness, and robust data protection. Additionally, the complexities of model governance require that AI models are interpretable and operable under clear guidelines.
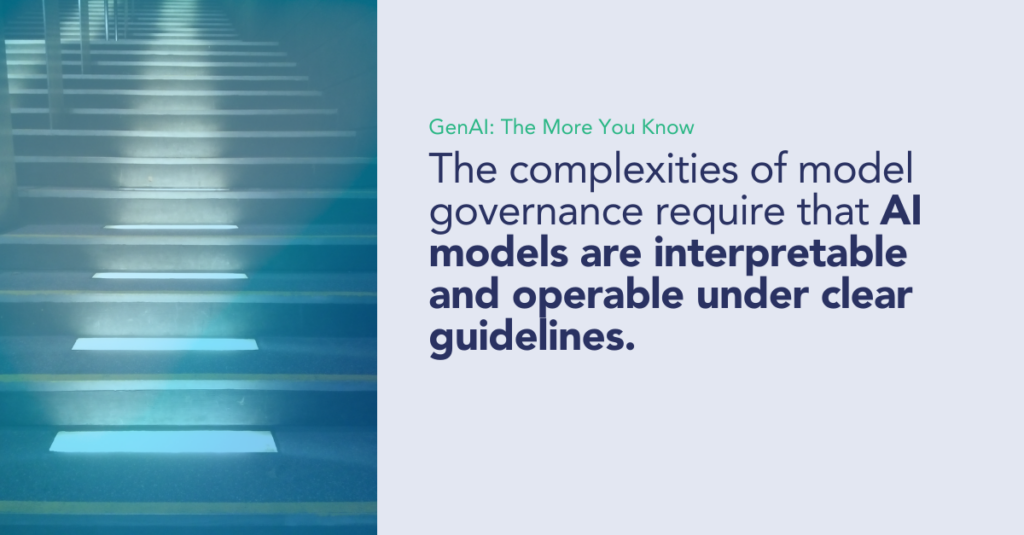
Operational compliance further compels adherence to stringent regulatory frameworks like GDPR and CCPA, which focus on privacy and data protection. Without continuous monitoring and auditing in these shadow environments, deviations in AI behavior cannot be promptly identified and rectified.
Strategically, the resistance to adversarial attacks highlights the vulnerability of AI systems to exploits that target model weaknesses, posing significant security risks. Moreover, the threat of data exfiltration through jailbreaking and other attacks exposes sensitive data, compounding the governance challenges.
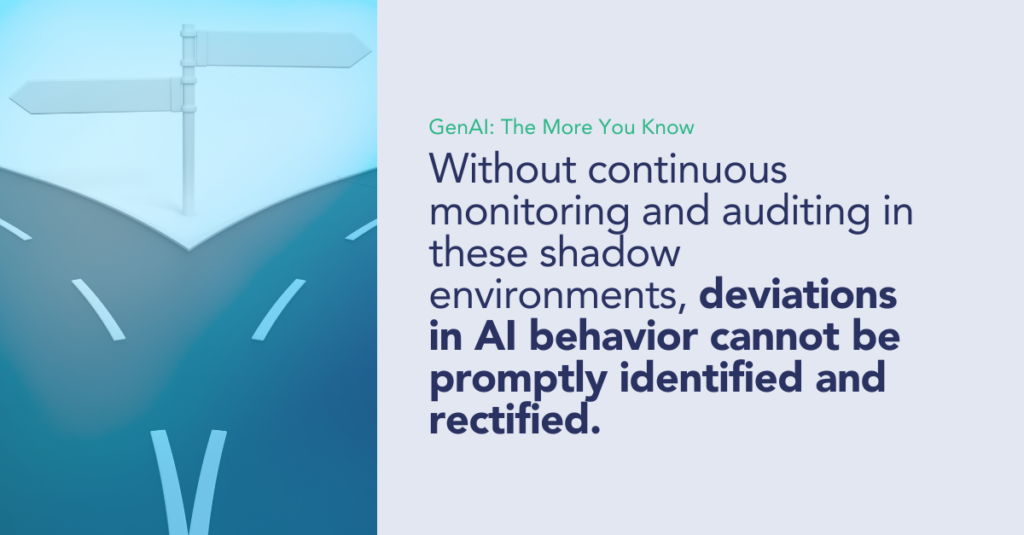
Operational Integrity & Ethical Concerns Surrounding Generative AI
Operational integrity and ethical concerns underscore the complexities of deploying generative AI technologies. Transparency is paramount, requiring clear documentation and traceability of AI decisions to facilitate audits and ensure regulatory compliance. The learning processes of AI systems present inherent risks, as sensitive input data may inadvertently be revealed or misused in outputs, epitomizing the “What goes in may come out” risk scenario.
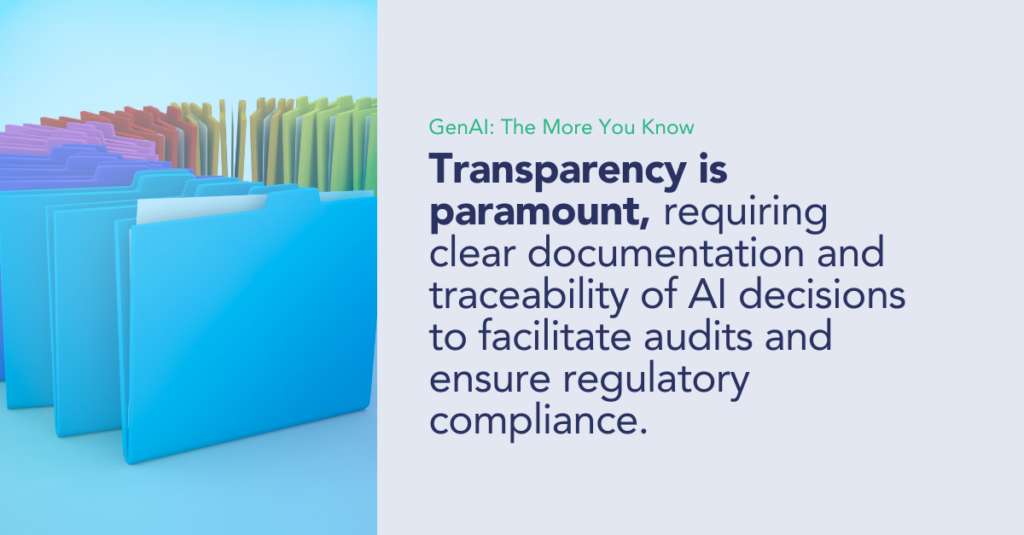
Furthermore, maintaining ethical and legal integrity is crucial. It is essential that AI-generated content respects copyrights and adheres to ethical standards, ensuring that digital creations do not infringe on existing intellectual properties or ethical norms. Additionally, there is a significant need for accountability in AI operations, particularly in addressing biases and ethical implications of AI decisions. This accountability is fundamental to fostering trust and maintaining the responsible use of AI technologies.
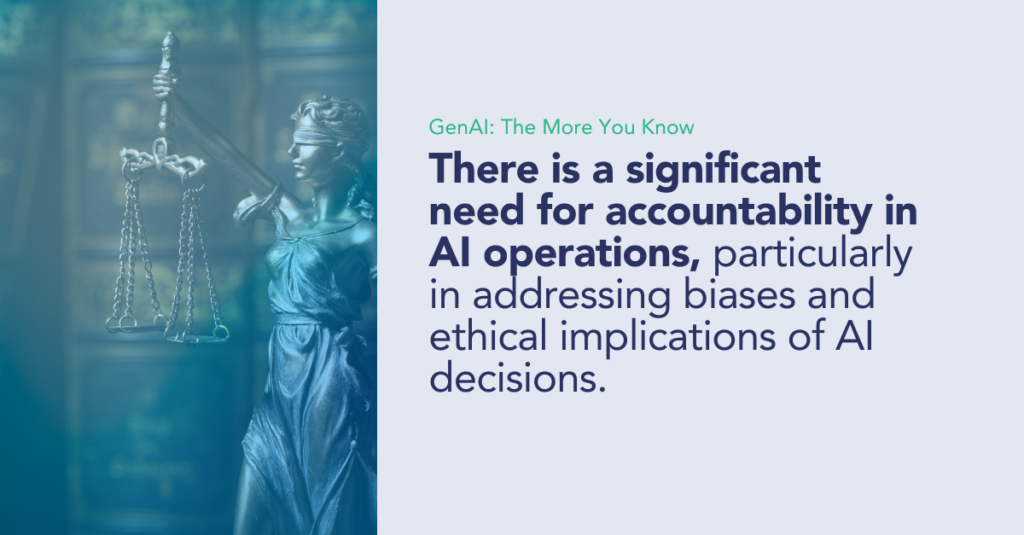
Protecting Privacy Across IT
Understanding the flow and storage of sensitive data is crucial for its protection within an organization. One such solution for this is the use of a Data Detection and Response (DDR) platform. DDR plays a key role by enabling organizations to comprehensively understand and sanitize their data before it crosses various boundaries. This process is instrumental in preventing the misuse of data and its unintended transfer to unauthorized or unmonitored IT environments, including Shadow IT systems and generative AI platforms. Organizations can significantly reduce the risks associated with data privacy and security breaches by ensuring that data is properly managed and sanitized.
DDR systems enhance protection against Shadow AI usage by providing comprehensive visibility into data movement within an organization. This visibility allows DDR to uncover Shadow IT systems, including unauthorized AI implementations often invisible to central IT departments. By monitoring these data flows, DDR can help prevent sensitive information from being inadvertently or maliciously processed by shadow AI systems, thus safeguarding the organization’s data integrity and compliance posture.
Advanced DDR manages data security by tracking how data is accessed and shared within an organization. This tracking capability enables DDR to identify unauthorized or accidental data flows to Shadow IT applications, including Shadow AI tools outside official oversight. By detecting these flows early, DDR allows for timely intervention, helping to prevent potential security breaches and ensuring that data handling complies with organizational policies.
DDR also enhances data security by enforcing consistent policies across all data interactions within an organization. This enforcement ensures that data within Shadow IT environments, which often operate without formal oversight, is handled according to the established organizational security standards. By applying these policies universally, DDR helps maintain the integrity and confidentiality of data, even in unofficial or hidden systems, thereby strengthening overall data governance.
How Votiro Covers Your Shadow Assets
Votiro’s advanced, Zero Trust DDR platform helps form a secure foundation for managing privacy across all your visible and Shadow IT. Using a Zero Trust architecture and proactive content disarm and reconstruction (CDR) capabilities, Votiro ensures that all data—in transit or at rest—is scrutinized and sanitized of potential threats and privacy risks before they can cause harm or be exposed to the wrong users. This approach helps ensure that sensitive data is monitored no matter what boundaries it crosses.
Votiro’s Zero Trust Data Detection and Response also enhances overall system resilience and data integrity, which is essential for maintaining compliance and safeguarding sensitive information.
Explore how Votiro can transform your security strategy and prepare your organization to manage its Shadow IT challenges by signing up for a one-on-one demo or by trying our platform free for 30 days.
News you can use
Stay up-to-date on the latest industry news and get all the insights you need to navigate the cybersecurity world like a pro. It's as easy as using that form to the right. No catch. Just click, fill, subscribe, and sit back as the information comes to you.
Sign-up Here!
Subscribe to our newsletter for real-time insights about the cybersecurity industry.